The allure of foundation models in medicine is undeniable. Foundation models are large-scale machine learning models trained on broad data at scale and designed to be adaptable to a wide range of downstream tasks. In natural language processing and computer vision, they’ve demonstrated remarkable capabilities. GPT-4, for instance, can generate human-like text responses, and models like CLIP can interpret and generate images based on textual descriptions. The success of these models is largely a function of the availability of massive amounts of data—text and images abundantly available on the internet. These models, built upon vast datasets and sophisticated architectures, promise to also revolutionize healthcare by predicting outcomes and personalizing treatments. But as we stand at the brink of a potential revolution, we must ponder: Are these models truly as powerful and reliable as people claim them to be, or are we being swept away by the tide of hype?
An observation from academic @lpachter on the social media platform X captures the doubt about this hype: “I don’t understand what the term ‘foundation model’ means… Is it just a catch-all phrase to signal ‘we did something similar to ChatGPT’?” This skepticism is healthy and necessary. It prompts us to question whether we’re adopting these models for their actual utility or simply chasing the allure of cutting-edge technology.
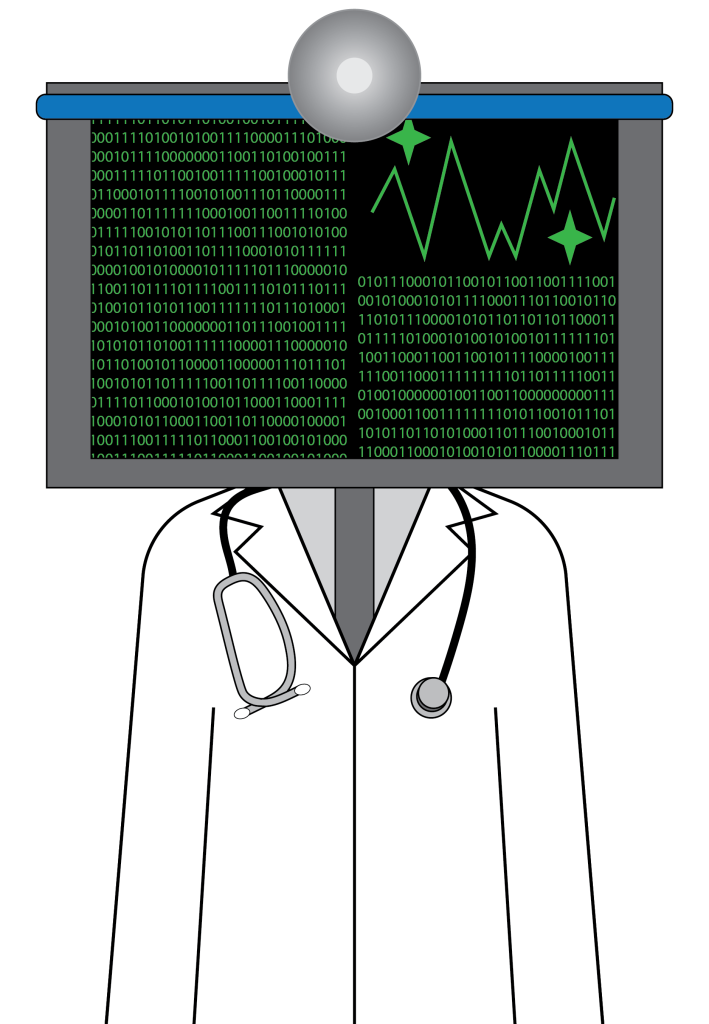
In recent literature, there has been a surge of interest in applying foundation models to biomedical tasks. A study titled “Deep learning-based predictions of gene perturbation effects do not yet outperform simple linear methods” highlighted a crucial point. Researchers benchmarked state-of-the-art foundation models, including transformer-based models and graph-based deep learning frameworks, against deliberately simplistic linear models in predicting gene perturbation effects. Surprisingly, a simple additive model outperformed a deep-learning counterpart for combinations of two gene perturbations, where only the data for individual single perturbations were available. For perturbations of genes not previously seen but potentially interpolated from biological similarity or network context, linear models performed just as well as deep-learning-based approaches.
This finding underscores a critical issue—complexity doesn’t always equate to performance. While deep neural networks hold promise for representing biological systems, there’s a need for critical benchmarking to direct research efforts effectively. Currently, many foundation models are not compared against simpler models or previous benchmarks, making it difficult to assess their true value. By systematically evaluating these models against established baselines, researchers can determine whether the added complexity offers a significant advantage or if more straightforward methods are good enough.
Dr. Fei Wang, Professor of Health Informatics in the Department of Population Health Sciences at Weill Cornell Medicine, emphasized that while the promise of foundation models is enticing, their success in medicine is limited by data availability and quality. “We see the models improving the larger they are in the general domain,” he noted. “But in biomedicine, we lack the scale and accessibility of data.” The application of foundation models in medicine involves a lot of complexities. Clinical data is inherently different from general domain data. It is sensitive, heterogeneous, and often siloed due to privacy concerns. Unlike the freely available data on the web, medical data requires stringent ethical considerations before being utilized. Generating the requisite volume of high-quality multimodal medical data (combination of images, genomics, text, etc.) is not only costly but also demands significant expertise and time.
From a philosophical standpoint, this raises questions about our approach to innovation. Are we attempting to force-fit a solution simply because it’s the latest trend? The hype around foundation models may, in part, stem from their success in other domains, leading us to believe they can serve as a silver bullet for complex medical problems. But medicine is not merely another data-rich field; it is a deeply intricate system where lives are at stake.
The success of such models hinges on several critical factors:
- Data Scale and Quality: Unlike general text or image data, medical data is not only scarce and often messy but also fraught with inconsistencies and riddled with missing data.
- Benchmarking and Evaluation: There is a lack of standardized benchmarks in medical AI. As Professor Wang mentioned, without rigorous comparisons to strong baselines, it’s difficult to ascertain the true performance of foundation models.
- Interpretability: The “black box” nature of deep learning models poses a significant barrier for interpretation in medicine. Clinicians need to understand the rationale behind predictions to trust and act upon them.
- Regulatory and Ethical Considerations: Deploying AI models in clinical settings requires compliance with stringent patient privacy and data security regulations, adding layers of complexity to the development and deployment of these models.
Dr. Quaid Morris, a professor at Memorial Sloan Kettering Institute, proposed that the true potential of foundation models in medicine lies in their ability to serve as powerful feature extractors. “At their best,” he suggested, “foundation models should be an interface for medical records, providing robust features for training extractors and predictors.” Rather than focusing on end-to-end clinical applications, these models could excel in downstream research tasks.
However, he also cautioned about technical challenges. “The drawback of foundation models is that they’re just too big,” he noted. Training and deploying such models require specialized hardware and significant computational resources, which can be a barrier for many academic institutions. Moreover, in some cases, simpler models or industry solutions might be more practical.
Dr. Olivier Elemento, Director of the Caryl and Israel Englander Institute for Precision Medicine, highlighted that while foundation models may show promise in research settings, there’s a significant gap in utility when it comes to clinical application. Retrospective datasets used in studies are often clean and curated, which is far from the reality of clinical environments. He emphasized the need for randomized controlled trials to validate these models in real-world settings, much like any new drug or treatment.
There’s a broader societal dimension to this discussion. The excitement around foundation models is part of a larger narrative about AI’s potential to transform industries. However, history teaches us that technological revolutions often come with unintended consequences. In the early days of genomics, there was immense optimism that sequencing the human genome would unlock cures for countless diseases. While it has led to significant advancements, the reality was more complex. Similarly, foundation models may not be the panacea for all medical challenges. It’s worth considering the concept of the “technological imperative” or the idea that if we can develop a technology, we should, and we must find ways to use it. This mindset can lead us to prioritize innovation over necessity, potentially diverting resources from more pressing needs.
In medicine, the ultimate goal is to improve patient outcomes. Every new tool or model should be evaluated through this lens. Are we enhancing care? Are we addressing unmet medical needs? Are we doing so ethically and sustainably? The intersection of AI and medicine is a journey of exploration. It is a path that requires both ambition and humility, innovation and caution. By grounding ourselves in rigorous science and ethical principles, we can navigate this landscape thoughtfully, ensuring that advancements truly serve the betterment of human health.