How does text-to-image AI work?
Artificial Intelligence (AI) has seen tremendous growth in the last two decades such that it is now starting to permeate most workplaces, especially with the recent open-source models like DALL-E and chatGPT. Science is no different.
AI uses machine learning algorithms that refer to the ability of a computer to find and learn data patterns without explicit instruction in ways that humans may not be able to. Most of the recent powerful machine learning algorithms try to mimic human neuronal networks, by creating elaborate connections between network nodes (mimicking neurons). Unlike the human brain, these neural networks learn via backpropagation. Backpropagation is a method with the goal of increasing accuracy of the neural network. This is accomplished by employing an algorithm that minimizes the error between the predicted and the actual results and then feeding that error optimization to the earlier steps of its algorithm. This is why computers need hundreds or even thousands of images to reduce their prediction errors.
The field is busy with developing different architectures that allow networks to learn more efficiently with fewer nodes. All of the recent AI natural language processing uses the transformer model architecture developed by Google. At the heart of the transformer is a multi-head, self-attention mechanism that weighs the information content of an input by considering the input’s context to adjust its influence on the output, i.e. the model uses the fact that word meanings depend on their context. Another important feature is that the outputs are generated in parallel, making training more efficient. AI image generators such as DALL-E extend the transformer’s capabilities to the domain of image generation. By training on a vast dataset of text-image pairs, the transformer learns which sequence of pixels (visual representation) best represents a scene (textual descriptions). However, the newer DALL-E 2 and 3 versions now use a method called diffusion, whereby image generation begins with a random field of noise which the neural network subsequently denoises to align the image to the interpretation of the prompt. Other models like Midjourney have all focused on the diffusion architecture in their most recent releases, as it shows the most promise.
The recent boom in AI is primarily due to the advances in computational power, data gathering and storage, and the use of large parallel computing platforms like graphics processing units (GPUs). However, it seems that critical debates about how such powerful tools can or even should be used by scientists cannot keep up with the rapid developments in the AI field.
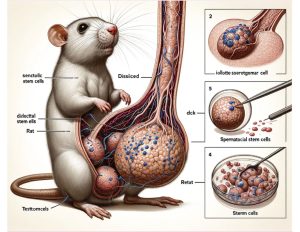
AI controversies in science
Using AI models is incredibly attractive to busy researchers, because it saves us lots of time in writing, editing, research, coding, debugging, and data visualization. Yet, there are a lot of concerns and unknowns about the accuracy of these models. Despite rapid and astonishing progress, generative AI tools still have critical limitations – they have a curious tendency to “hallucinate” and produce confabulations with high confidence, such as referencing non-existent publications, generating false statements and incorrect images.
Such mishaps led to a recent controversy with a paper published in Frontiers in Cell and Developmental Biology on February 14th, 2024. The paper reviewed the JAK/STAT signaling pathway in spermatogonial stem cells and included an obviously AI-generated, awkward, if not grotesque, image of rat testes and a collection of other scientific-looking diagrams with little to no accurate scientific content. Even the labels had terrible spelling errors or were complete gibberish. Any biologist would realize how ridiculous and meaningless such images are within seconds, but the editors didn’t find the images troubling enough to reject it in review. Once published, the paper was soon retracted for “concerns [that] were raised regarding the nature of its AI-generated figures”. This brought up several questions about the use of AI generated images in science and the potential issues of peer review.
How this paper passed peer review to be published is still a question, but the leading consensus seems to be that it was not reviewed at all. While this case was caught quickly post-publication, there are likely many more that have slipped by with problems that are trickier to catch. We do not yet have proper tools to check the integrity of AI-generated work and differentiate between the legitimate and the fabricated, so we need to rely on a robust peer review process instead. Currently, many instances of research misconduct in the submission, peer-review, and editing stages occur because of an inadequate level of human attention to detail. Issues go unnoticed because they receive too little scrutiny from authors, editors, and reviewers.
Community response to AI images
AI technology is rapidly developing, so the policies directing its use in scientific work and publishing need to keep up with the new ways in which AI images and text are being used. While most journals agree that using AI-generated text does not warrant attributing authorship to the language model, generative AI images raise new kinds of copyright issues and research integrity concerns. Legal issues surrounding AI-generated material are broadly unresolved and not universally accepted. Fortunately, some journals have already made explicit instructions on how or when such material can be used and how it is to be reported, even long before the Frontiers paper controversy.
Springer Nature and the Science family journals do not allow AI-generated images in their publications, with the exception of publications specifically relating to AI and images explicitly permitted by the editors. A violation of these policies constitutes scientific misconduct considered no differently from manipulation of data images or plagiarism of existing works. Springer’s policy does permit AI-generation of text– and numerical-based materials such as tables, flow charts and simple graphs. Meanwhile, the World Association of Medical Editors (WAME) recommends authors to provide the full prompt, the date and time of the query and the AI tool used when reporting AI-generated tables, figures or code. PLOS ONE follows a similar principle, allowing AI images only if the authors declare the AI tool, the query, and how the authors verified the quality of the generated content. The International Committee of Medical Journal Editors (ICMJE) also urges journals to require disclosure of AI use in both cover letters and submitted work. The ICMJE also encourages journals to hold authors responsible for ensuring the generated work does not constitute plagiarism. Similarly, the Council of Science Editors (CSE) also recommends that journals enforce policies about the use of AI-generated images and ask authors for the technical details of the model and the query used.
Where does the Tri-I stand?
Within our Tri-I community, a multidisciplinary task force at Cornell issued a report in January 2024 offering perspectives on generative AI and practical guidelines for its use in academic research for experimental conception, execution and dissemination, as well as funding proposals, funding agreement compliance and translation of research work to copyrights or patents. The poor information quality and accuracy resulting from AI hallucinations, biases or bugs were of great concern. Some of the risks highlighted in the report include poor oversight of compliance that could lead to financial, regulatory, legal, and reputational consequences for the institution and the individuals involved. The reputational damage of improper AI use could extend beyond institutions to impact funding organizations, community dissatisfaction, public relations, and faith in scientific publishing as a whole.
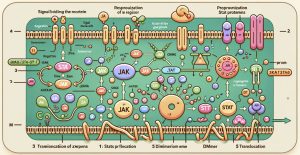
Where do we go from here?
As it stands, only the authors are accountable for all aspects of a manuscript including the accuracy of the content that was created with the assistance of AI, regardless of how many other people may have been involved in facilitating the publication. The controversy surrounding the Frontiers paper lies in the fact that the manuscript passed review without any of the reviewers or editors catching the glaring scientific inaccuracies. At a time when trust in science is eroding due to the reproducibility crisis and political attacks on scientists’ trustworthiness, it is important for scientists to recommit to careful and meticulous attention to detail. This is especially true for those put in charge of determining what kind of science gets published; these publication decisions often determine the opportunities scientists have to advance their careers. As AI models become more advanced and harder to distinguish from human work, regulation could play an important role in determining what kind of scientists we will be in the future.